Unlocking Stability: The Power Of A Positive Definite Matrix
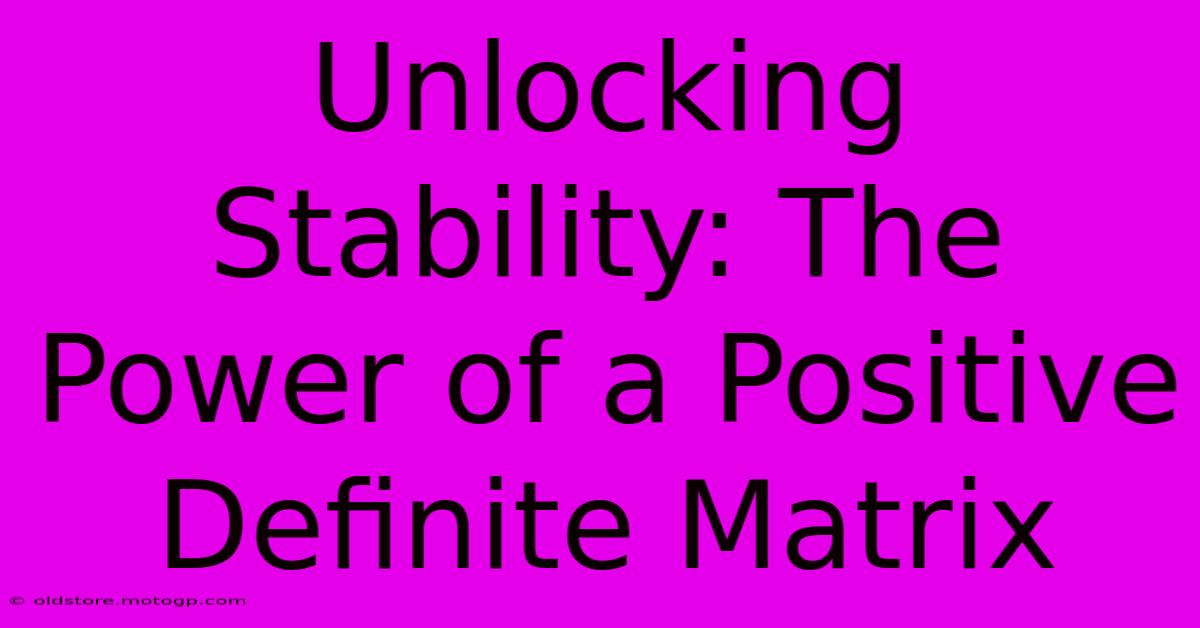
Table of Contents
Unlocking Stability: The Power of a Positive Definite Matrix
Positive definite matrices are fundamental in numerous fields, from engineering and physics to machine learning and finance. Understanding their properties is crucial for solving complex problems and ensuring stability in various systems. This article delves into the core concepts of positive definite matrices, exploring their characteristics, applications, and significance in guaranteeing stability.
What is a Positive Definite Matrix?
A positive definite matrix is a symmetric square matrix where all its eigenvalues are positive. Equivalently, for any non-zero vector x, the quadratic form x<sup>T</sup>Ax is positive, where A is the matrix in question. This seemingly simple definition has profound implications for the stability and well-behavedness of systems represented by these matrices.
Key Characteristics of Positive Definite Matrices:
- Symmetry: A positive definite matrix is always symmetric (A = A<sup>T</sup>). This symmetry is essential for the existence of real eigenvalues.
- Positive Eigenvalues: All eigenvalues (λ<sub>i</sub>) of a positive definite matrix are strictly greater than zero (λ<sub>i</sub> > 0).
- Positive Definiteness of Submatrices: All principal minors (determinants of submatrices obtained by deleting rows and columns with the same index) are positive. This provides a practical method for checking positive definiteness.
- Cholesky Decomposition: Positive definite matrices can be uniquely decomposed into the product of a lower triangular matrix (L) and its transpose (L<sup>T</sup>): A = LL<sup>T</sup>. This decomposition is crucial for efficient computations.
Applications of Positive Definite Matrices:
The applications of positive definite matrices are vast and span diverse disciplines:
1. Optimization and Machine Learning:
- Convex Optimization: Positive definite matrices are essential in convex optimization problems. The Hessian matrix (matrix of second-order partial derivatives) of a convex function is positive definite, guaranteeing a unique global minimum. This is fundamental in algorithms like gradient descent.
- Covariance Matrices: In machine learning, covariance matrices, representing the relationships between variables, are always positive semi-definite (a broader class including zero eigenvalues). Positive definiteness indicates linearly independent variables.
- Kernel Methods: Kernel functions used in Support Vector Machines (SVMs) and other kernel methods often generate positive definite matrices, ensuring the validity of the algorithms.
2. Engineering and Physics:
- Structural Analysis: In structural mechanics, the stiffness matrix representing the relationship between forces and displacements is positive definite, signifying structural stability.
- Stability Analysis of Dynamical Systems: Positive definiteness is a key criterion for checking the stability of linear dynamical systems. The Lyapunov stability theorem utilizes positive definite matrices to assess system stability.
- Electromagnetism and Quantum Mechanics: Positive definite matrices appear in various contexts in electromagnetism and quantum mechanics, often representing energy or probability distributions.
3. Finance and Economics:
- Portfolio Optimization: Covariance matrices of asset returns are used in portfolio optimization to minimize risk and maximize returns. Positive definiteness is crucial for the validity of Markowitz portfolio theory.
Ensuring Stability through Positive Definiteness:
The significance of positive definite matrices lies primarily in their role in ensuring stability and well-behavedness of systems. For example:
- Stability of Equilibrium Points: In dynamical systems, a positive definite Lyapunov function guarantees the stability of an equilibrium point.
- Convergence of Optimization Algorithms: The positive definiteness of the Hessian matrix ensures the convergence of many optimization algorithms to a global minimum.
- Well-conditioned Systems: Positive definite matrices often lead to well-conditioned systems, making numerical computations more reliable and less susceptible to errors.
Conclusion:
Positive definite matrices are far more than just a mathematical concept; they are powerful tools with extensive applications across various scientific and engineering fields. Their crucial role in guaranteeing stability, enabling efficient computations, and ensuring the validity of numerous algorithms underscores their importance in modern science and technology. Understanding their properties is a crucial step for anyone working with optimization, machine learning, or systems analysis. Further exploration into their specific applications within your chosen field will undoubtedly unlock even greater insights into their power and utility.
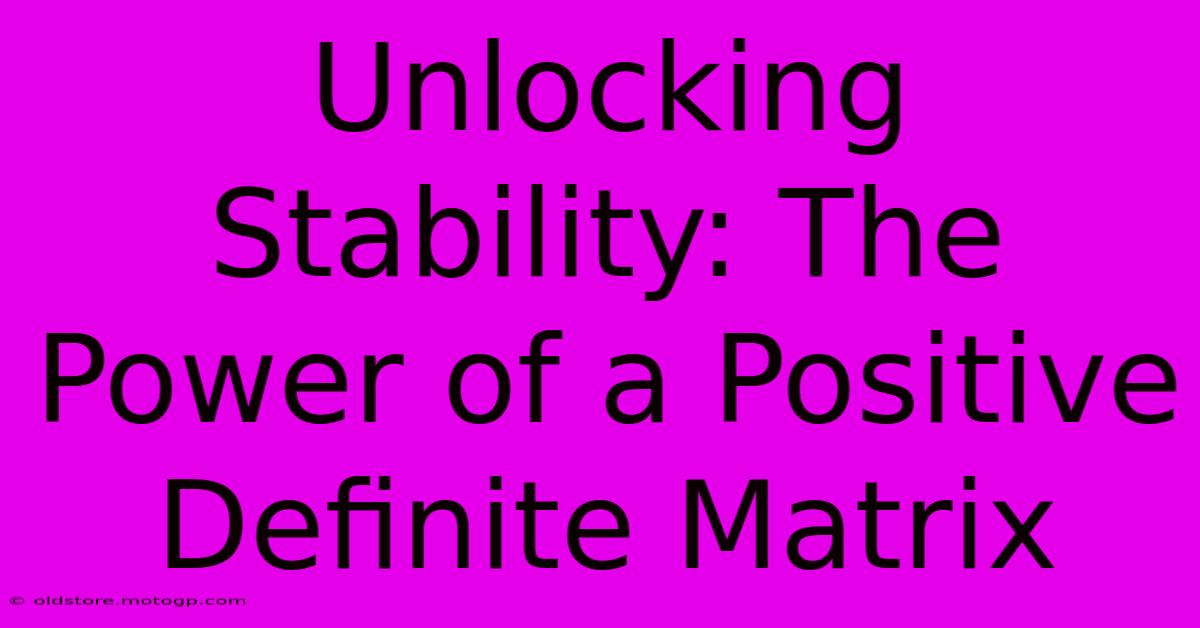
Thank you for visiting our website wich cover about Unlocking Stability: The Power Of A Positive Definite Matrix. We hope the information provided has been useful to you. Feel free to contact us if you have any questions or need further assistance. See you next time and dont miss to bookmark.
Featured Posts
-
Diego Klattenhoff More Than Just Homeland Discover His Incredible Range
Feb 14, 2025
-
Overcome Any Obstacle Glindas Wisdom For Modern Challenges
Feb 14, 2025
-
Quick Tip Convert 70 Mph To Kmh In Seconds
Feb 14, 2025
-
Plan Your Perfect Getaway Dundas Castle In Roscoe Ny
Feb 14, 2025
-
Our Lady Of The Rockies Awe Inspiring Views And Inner Peace
Feb 14, 2025